The realities of emerging AI/Machine learning healthcare products
Read in Magazine PDF
he term Artificial Intelligence (AI) is a broad category that covers many computing methods intended to mimic or reproduce human learning and decision-making. The goals of AI programs for healthcare are multifold for clinicians and/or patients, including enhancing clinical decision-making, reducing medical errors, reinforcing quality and safety practices, and optimizing healthcare processes to reduce waste and increase satisfaction by all stakeholders. The need and pressure for designing and deploying AI tools to augment and improve patient care, safety, and satisfaction is growing rapidly because ageing populations and constrained clinician labour pools threaten to overwhelm many hospitals, clinics, and practices.
This article is divided into three parts: 1) Overview of terminology; 2) Successful healthcare AI examples; 3) Realities, Limitations, and Challenges. Readers with prior AI knowledge may choose to skip Part 1, but may refer back to it when clarification is desired.
Part 1a:
There are two broad AI categories deployed in healthcare: rule-based (RB) and machine learning (ML). RB systems are typically the simplest because they are based on relatively structured known clinical and scientific facts. e.g., if a patient’s blood pressure is greater than 130/70 mmHg, according to the American Heart Association’s 2017 rubric treatment for high blood pressure (hypertension) could be indicated. RB AI runs into complications, though, when patients have multiple diseases (multi-morbidities), because, for example, drug-drug (or drug-treatment or treatment-treatment) interactions, disease-drug interactions, and patient-specific undesirable drug side effects and risks are interdependent.
For example, the same 130/70 mmHg finding may have a different interpretation and treatment pathway for an otherwise young, fit active adult compared to an obese senior with diabetes and shortness of breath. In such cases, a category of fuzzy-logic programs may be used to supplement RB AI systems. Fuzzy logic may also be deployed in RB systems for radiology, pathology, or similar image analysis, assisting detection of difficult-to-discern tumours or other anatomic irregularities or injuries. Fuzzy logic calculations move past binary yes-no or true-false decision branching using statistical estimates to help move “maybe” cases into yes or no paths.
Part 1b:
The second broad class of AI, Machine Learning (ML), is characterized by programs that “learn,” typically using prior or current data to diagnose, prescribe, or predict healthcare situations. e.g., determining if a chest CT image suggests COVID-19, flu, or pneumonia, deciding which drug prescription regimen would offer the best risk/benefit tradeoff, or triaging incoming COVID-19 patients to predict and identify those who are at the highest risk of serious fatal complications. One may encounter numerous ML methodological terms and applications, including Artificial Neural Networks (ANN), Natural Language Processing (NLP), Bayesian Systems, and Ensemble methods. ANNs use programs to simulate the human nerve process of summing multiple sensory inputs – i.e., digitized human data — over time to trigger a response.
NLP may use a combination of ANN, filters, RB libraries, and context clues to translate spoken words into correct phrases or codes. Bayesian-based programs may use an iterative process of error detection and success seeking. Ensemble methods may combine multiple RB and ML tools in parallel to identify the most accurate tool for a situation. A further ML categorical split exists between trained ML and self-learning ML tools.
In trained ML, the program’s learning is derived from human experts Part 1b: The second broad class of AI, Machine Learning (ML), is characterized by programs that “learn,” typically using prior or current data to diagnose, prescribe, or predict healthcare situations. e.g., determining if a chest CT image suggests COVID-19, flu, or pneumonia, deciding which drug prescription regimen would offer the best risk/benefit tradeoff, or triaging incoming COVID-19 patients to predict and identify those who are at the highest risk of serious fatal complications. One may encounter numerous ML methodological terms and applications, including Artificial Neural Networks (ANN), Natural Language Processing (NLP), Bayesian Systems, and Ensemble methods. ANNs use programs to simulate the human nerve process of summing multiple sensory inputs – i.e., digitized human data — over time to trigger a response.
NLP may use a combination of ANN, filters, RB libraries, and context clues to translate spoken words into correct phrases or codes. Bayesian-based programs may use an iterative process of error detection and success seeking. Ensemble methods may combine multiple RB and ML tools in parallel to identify the most accurate tool for a situation. A further ML categorical split exists between trained ML and self-learning ML tools. In trained ML, the program’s learning is derived from human expert annotated training sets. e.g., libraries of curated heart arrhythmias. The quality of the final trained ML is determined by assessing its precision and errors (false negatives or positives) using a different set of known, annotated heart arrhythmias.
By contrast, self-learning ML programs evaluate each new piece of data against prior data patterns that, optimally, result in self-organizing clusters. Those clusters can then be assigned meaning by experts. e.g., one cluster may represent patients who have severe pulmonary long-COVID symptoms versus another cluster of patients with severe neurologic long-COVID symptoms.
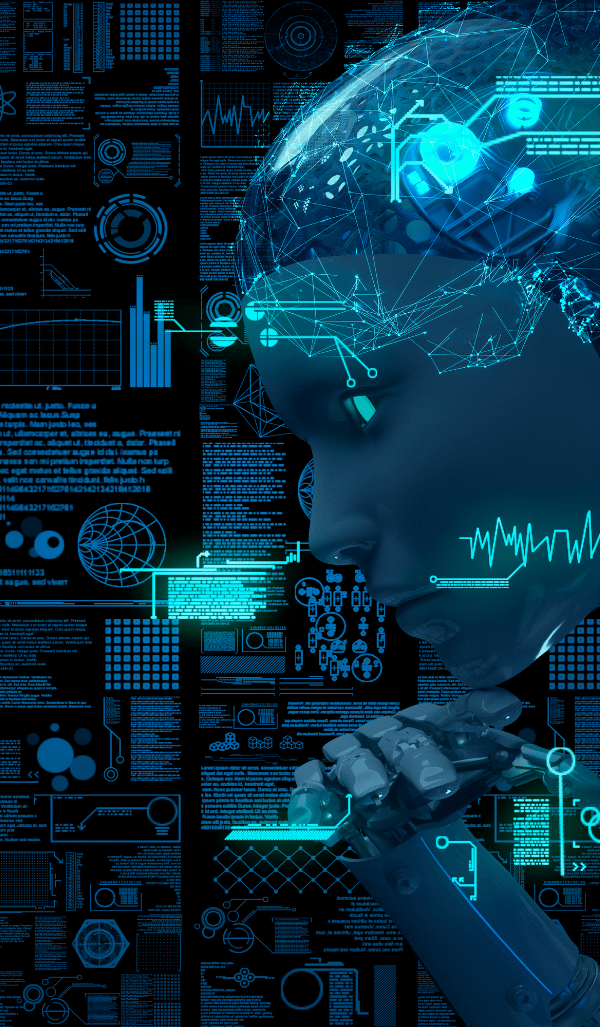
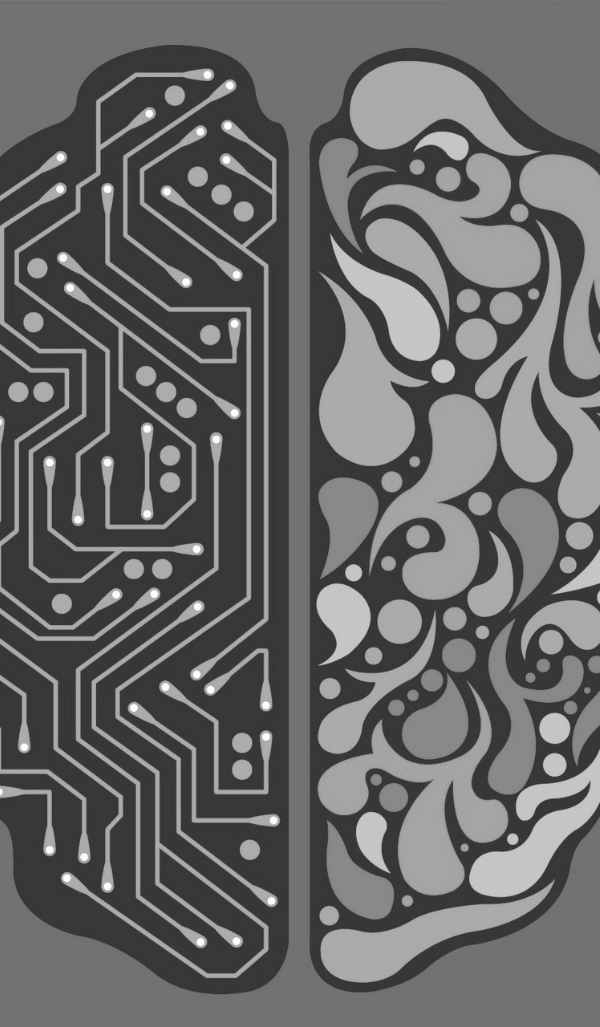
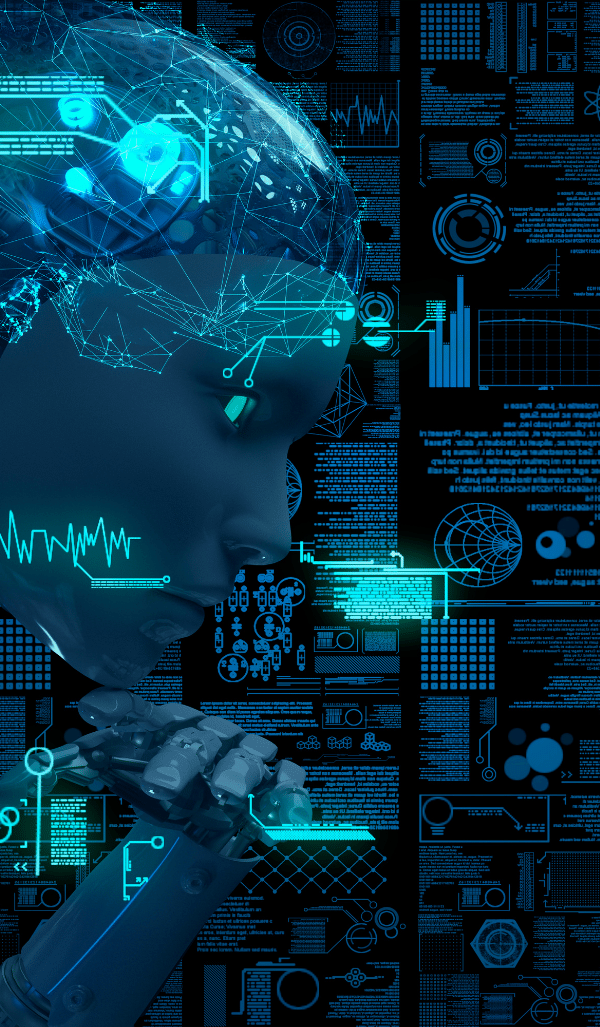
Part 2:
Some examples of successful AI in healthcare can be derived from the COVID-pandemic literature. The UK Pulse Oximetry at Home and Virtual COVID Ward programs are examples of RB systems, and similar programs have emerged in the US and elsewhere. By contrast, many articles in the radiology and ultrasound imaging literature describe emerging and applied lung and organ imaging ML methods to identify, confirm, or disambiguate COVID-19 when other symptoms or lab diagnostics are inconclusive or contradictory. Another exploratory COVID-19 ML application with a mixed track record has been COVID case and hospitalization forecasting.
Other AI applications were already being developed and deployed in the decade before the pandemic. Examples include NLP for clinician dictation in discrete fields like radiology or pathology, Clinical Decision Support Systems (CDSS) that help match cancer treatments to individual genomic, proteomic, and genotypic data, radiologic and pathologic image tumour identification and second-opinion creation, and matching patient populations to clinical trial requirements and goals. Exploratory ML applications include attempts to identify early stages of tumours, sepsis, dementia, or patient fall risks.
Part 3:
Realities, Limitations, and Challenges of AI in Healthcare. One needs to acknowledge the successes that AI has had, as described in Part 2 above. Nonetheless, several significant limitations must be understood and accepted.
Part 3a:
First and foremost may be bearing in mind that AI is, at best, a simulation of human intelligence. There are no flesh-and-blood nerves, organs, emotions, or values in the programs – at least not yet. AI software perceives and analyzes a set, or many sets, of digitized patterns based upon prior imprinted data. A computational assessment of a patient’s vital signs, for example, cannot discern critical telltale signs that a physician, nurse, or caregiver would perceive, such as poor pallor, sad demeanour, cognitive confusion, glazed eyes, wincing facial pain, or unsteady gait. A computer has no compassion or empathy engine, either, so recommending complex and risky treatments for a very elderly patient with well-advanced Alzheimer’s disease may not seem unreasonable to the program.
Part 3b:
Verification, validation (V&V), and change management are significant challenges, too, because of the potential life, death, cost, and satisfaction consequence of errors. In most medical technologies, V&V requires a) verification the product does what was designed to do, and b) validation that it works properly for the intended application. So an AI program that shows high reliability for breast cancer detection in a population of North American women based on images collected from the latest generation of low-dose mammography devices may not produce valid results when examining a community of petite women with relatively small breasts using a 15-year old mammography device.
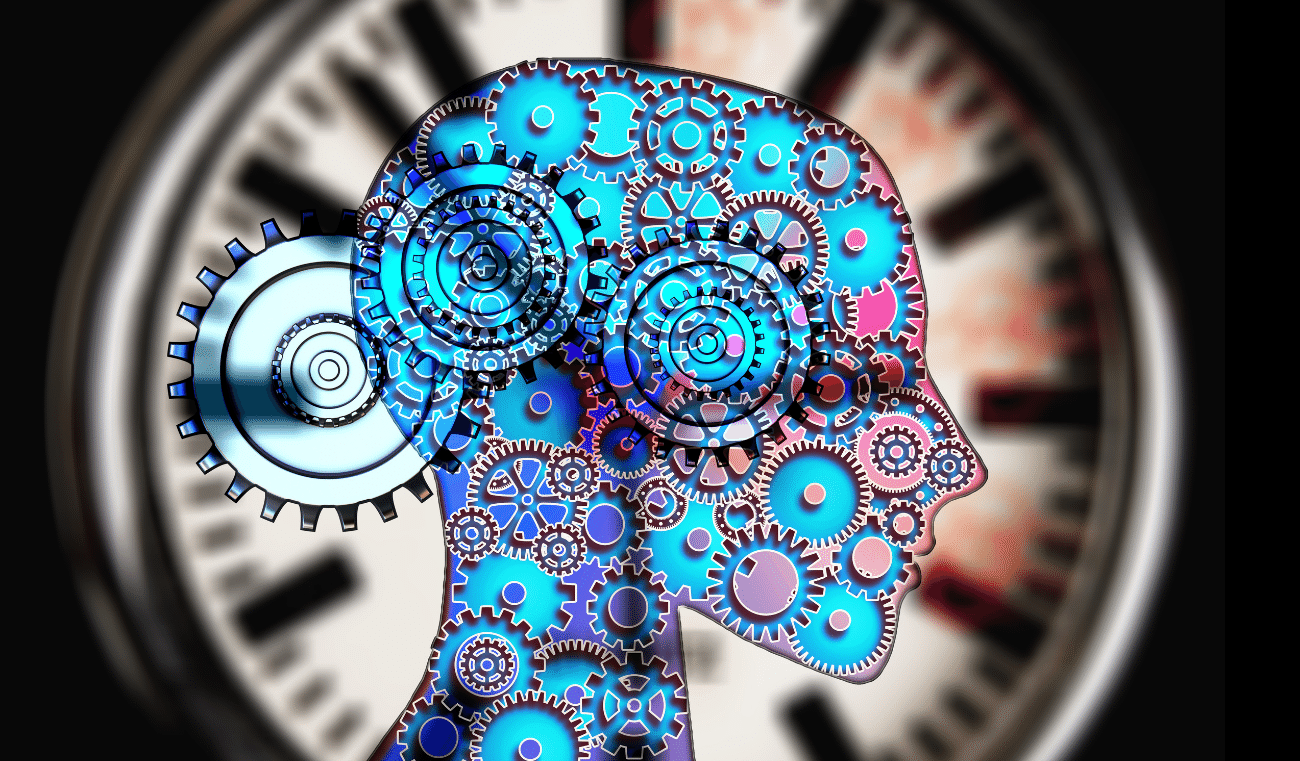
Further, since all software is eventually enhanced, updated, and repaired, defining and executing a reliable V&V process can be quite complex and may, in some cases, require some clinical trials. A senior colleague working in a very large global commercial healthcare AI endeavour described the unsatisfactory results they were having when attempting to apply carefully developed and validated AI systems that were curated from world-renowned speciality hospitals to world-class hospitals in diverse countries. Terminology, diagnosis, treatment practices, and available therapies were so radically different that satisfactory results could not be obtained and sustained.
Part 3c:
Because scientific and medical knowledge is constantly changing, re-training, and V&V of an AI system to incorporate significantly different new facts may be quite difficult. A human adopts new patterns (habits) by not only learning new facts and actions but also by suppressing or forgetting prior ones that are no longer believed to be correct. In the blood pressure/hypertension example in Part 1 above, when the American Heart Association reduced the hypertension threshold from 140 to 130mmHg, forcing an ML AI system to unlearn 140mmHg when it has already included data from 100,000 patient visits over 5 years may be considerably harder than simply changing a single rule in an RB AI system. ML systems are not typically designed with an “unlearn” or “forget” subsystem! An example from the COVID-19 pandemic may illustrate this challenge: at the onset of emergency cases in several cities in Italy and the US, the “best practice” for patients with acute respiratory distress was ventilator care in an ICU. That led to a massive effort to locate, commission, invent, and approve large numbers of ventilators, train clinicians to operate those ventilators and repurpose hospital beds for ICU care wherever possible. Unfortunately, COVID patients’ ventilator mortality rates were so astonishingly bad that by the end of the first wave, ventilators were no longer the care pathway of choice.
High-flow nasal oxygen cannulas, prone positioning, and even watchful waiting were found to have better survival rates. In such a crisis, with rapidly changing facts and discoveries, overriding an ML system or CDSS system would probably be far better than wasting time trying to re-train it!
Part 3d:
Transparency and interpretation of AI decision-making can erode clinician and patient trust. In the literature, “self-explaining” AI programs may sound preferable, but that may be a tall order for ML software. Even if the initial software was trained with a very well-annotated expert data set, such software may not be able to identify and disclose the way many nuanced changes and updates that occurred over many months or years which were based on, perhaps, many tens of thousands of new patient cases.
Part 3e:
By extension of the above Part 3 issues, the legal liability of AI software and AI software vendors is an emerging issue. If a patient is harmed, who is liable? Who is sued? Who has responsibility for the chain of events leading to harm? At one extreme, in the US, medical technologies are always presumed to be operated and under the control of a “learned intermediary,” e.g., the licensed physician, nurse, or allied health professional. However, if the intermediary’s decision-making was misinformed or misled by a software, sensor, or data deficiency, then interpreting and assigning culpability may be very complicated. Further, in the event of significant harm, incident and forensic investigation may require the preservation and examination of many years of software changes, V&V, and training records to understand the root cause. (The Boeing 737 Max crash debacle might be an example of the complexity.)
PART 3F:
AI is primarily a methodology to optimize the “as-is” state of medicine. In other words, the data that is collected to develop RB or ML AI reflects the clinical care pathways and processes that exist today. e.g., at the beginning of the pandemic, ICU admission and ventilator care were the well-documented and expected standard of care. Extreme rates of patient mortality could only represent situational anomalies in an AI system. To generate future “to be” states requires different tools, among which Simulation and Modeling (SM) stand out. With SM, a model of the new process flows can be developed based, in part on existing data and simulations of planned new pathways. e.g., some less acute patients might be routed to prone positioning beds, some relatively stable may be maintained in wait-and-see wards, and only those patients with high-survival-likely respiratory distress may be routed to ventilator care. If an existing AI system already is helping manage this latter group of ventilator patients, then the SM tool can help assess staff and facility requirements based on analyzing prior patient admission data. Estimates of the length of stay, staff ratios, equipment and supply needs, etc, can be modelled in the SM tool, helping to design an optimized sustainable model. As each new program begins to take and manage patients, new data can begin to accrue to help develop and validate new AI tools to support those care pathways. Thus, AI and SM can be seen as synergistic partners to support evolving patient care.
Conclusion:
There is little doubt that the ageing global population which often has multi-morbidities, combined with global clinical staff shortages all make the development and deployment of AI software tools very attractive. As in all medicine, there are complex risks and benefits. Clinicians and hospitals that wish to make use of AI technologies must carefully and prudently assess, deploy, and manage AI tools, always keeping a careful and vigilant eye on safety, efficiency, efficacy, and potential risks and limitations.
Authors
-
PhD, CCE Health Informatics Faculty and Research, Villanova University Certified Instructor, HIMSS CAHIMS, CPHIMS, & CPDHTS Programs Vice President, Foundation for Living, Wellness, and Health
-
PhD, CCE, FHIMSS, FAIMBE Health Informatics Faculty and Research, Villanova University Certified Instructor, HIMSS CAHIMS, CPHIMS, & CPDHTS Programs President, Foundation for Living, Wellness, and Health