The Safety and Effectiveness of Electronic Decision Support to improve care decisions and outcomes
Read the Magazine in PDF
Abstract
The use of electronic decision support systems (EDSS) in healthcare offers the potential for improved patient safety and efficiency. This article addresses EDSS benefits, limitations, individual and contextual factors affecting effectiveness, and future research and implementation directions. It emphasizes interdisciplinary collaboration, system customization, and continuous evaluation for successful EDSS implementation.
Introduction
Electronic decision support (EDS) involves using technology like computer software or mobile apps to help individuals or teams make informed decisions in various fields. In healthcare, EDS assists clinicians in diagnosis and treatment decisions by providing access to patient data and evidence-based treatment guidelines. EDS is broadly classified into two categories: Basic decision support and tools that focus attention. Advanced decision support: Tools that aim to provide patient-specific recommendations [1, 2].
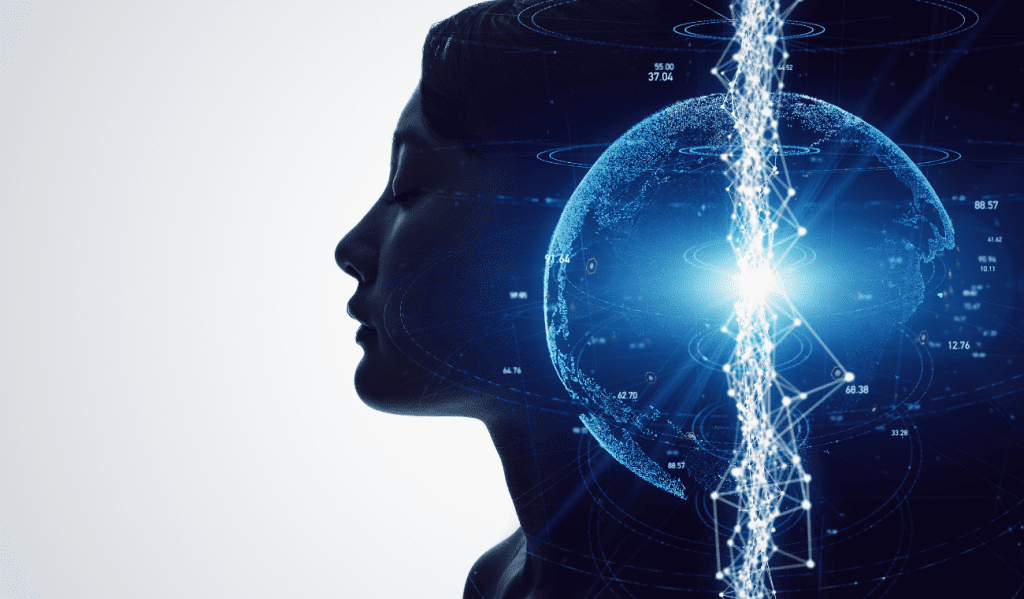
What is the best way to display decision support for healthcare providers using our electronic health record system?
Decision support can be classified into two categories:
- Passive decision support: Passive decision support presents information to the user that they must take action to access.
- Active decision support: Active decision support is presented in the form of electronic alerts that interrupt the user in their task. However, excessive use of alerts can interrupt clinical work and cause frustration, commonly known as “alert fatigue.”
Impact of decision support on repeat laboratory test ordering rates:
EDS in pathology test ordering helped reduce duplicate tests by introducing alerts to inform clinicians if a test had already been ordered. Success stories showcase how EDS can improve efficiency and prevent unnecessary repeat testing in healthcare settings [3].
Alert fatigue refers to a mental state resulting from excessive numbers of alerts being triggered and ultimately leads to:
- User frustration,
- Overwhelmed prescribers,
- Ignoring all alerts.
Research indicates clinicians override a high percentage (49% to 96%) of medication alerts, making them ineffective.
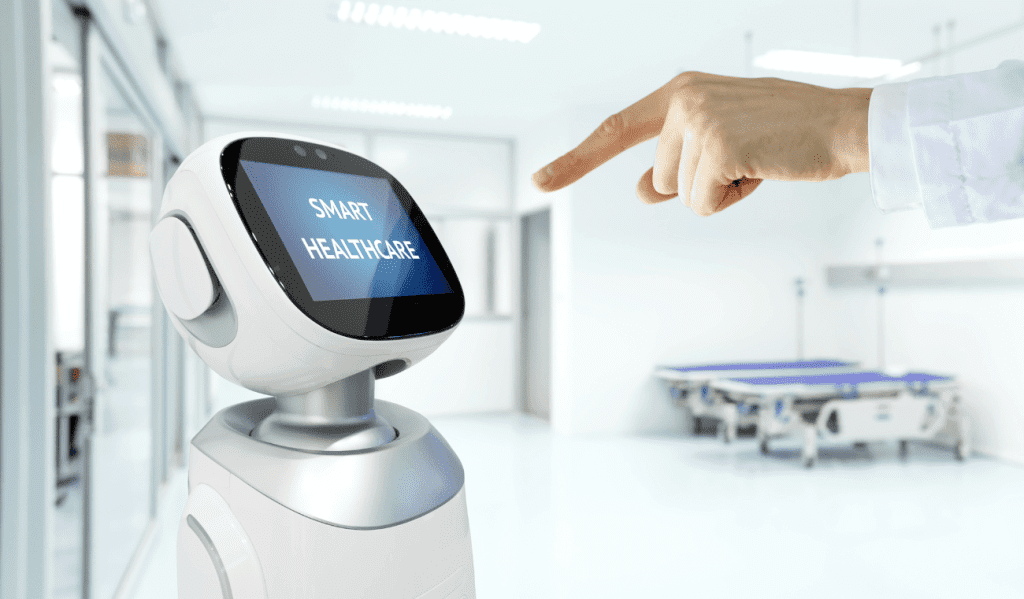
Design is Critical
Design is a critical factor in the success of decision support alerts. Poor design can lead to confusion and frustration among clinicians, rendering the alert ineffective.
There are several factors to consider when designing decision support alerts, including:
- Content: Clear, concise, and actionable.
- Visual design: Easy to understand and scannable.
- Tone: Respectful and non-judgmental.
- Context: The clinician’s workflow and patient’s clinical status.
Explanation of poor and good design in decision support alerts:
- Poor design: Difficult to understand at a glance or requires extensive reading and interpretation.
- Good design: Uses color and images to convey the seriousness of the issue and is easy to understand and act upon.
How do we optimize decision support alerts?
To optimize decision support alerts, hospitals must employ a combination of strategies, including feedback from clinicians, analyzing alert performance, and redesigning alerts as needed [4].
The following strategy can be adopted:
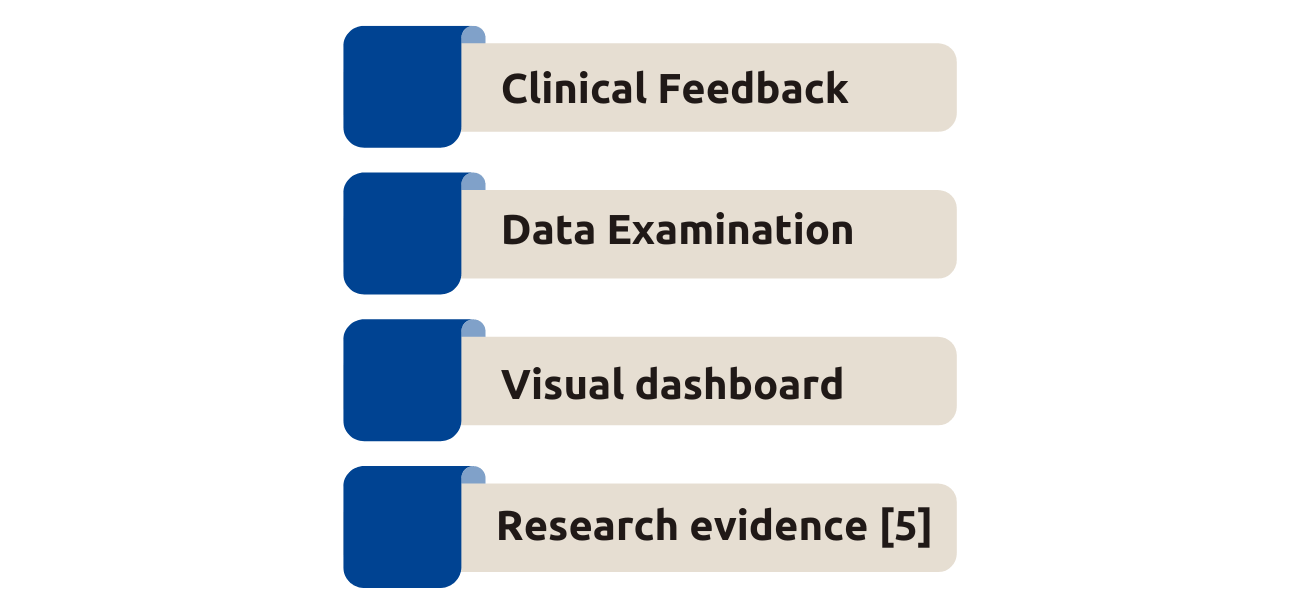
Use of Technology in Healthcare
The increased use of alerts in electronic health record systems has raised concerns about alert fatigue among clinicians. There is a growing need for an evidence-based approach to designing and implementing signals to address this. A study on drug-drug interaction (DDI) alerts revealed that while around one-third of hospitalized patients experience a DDI, only about 2% are harmed, suggesting the need to carefully weigh the benefits and risks of incorporating such DDI alerts into an Electronic health record system [6,7].
Assessing the impact of adding DDI alerts:
- One approach to assessing the potential impact of alerts is to first introduce alerts into a test system with a sample of ‘real’ hospital data and to determine the rate at which the new alerts would fire if added to the current hospital system.
- This process also allows alert to be refined prior to introduction to a current hospital system.
A study found in Australia used this approach. They found that adding DDI alerts to an existing electronic health record system in a hospital would significantly increase the number of alerts received by clinicians, highlighting potential risks and the need to consider the impact on clinicians before implementation.
Electronic Decision Support can offer real-time advice to improve patient safety and outcomes, but careful consideration of the potential risks, including alert fatigue and information overload, is essential during implementation.
The potential benefits of EDS are significant and include
- Improved patient safety,
- Reduced medication errors,
- Increased efficiency,
- Cost-effectiveness.
- The benefits of EDS must be balanced against potential risks like alert fatigue and unintended consequences. System design, alert type, timing, and clinician characteristics can influence the system’s effectiveness and acceptance.
In a University of Michigan study, attending physicians showed higher acceptance and responsiveness to DDI alerts than resident physicians, emphasizing the need to consider individual factors in designing EDSS [8,9].
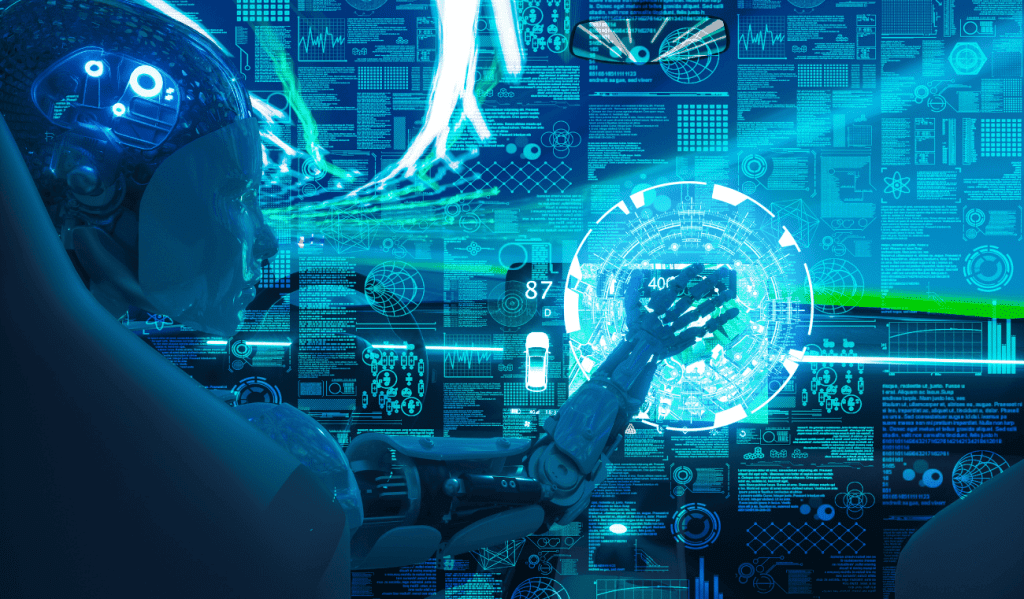
Strategies for Successful Implementation
To ensure the successful implementation of EDSS, it is important to consider both the benefits and risks and to develop strategies to balance these factors. Some potential strategies include:
1. Tailoring alerts to individual clinicians and patients,
2. Using evidence-based alerting criteria.
Design decision support systems that are effective in these high-pressure environments
The design of alerts significantly influences clinicians’ response rates, as evidenced by a study in the American Medical Informatics Association’s journal.
Alerts providing actionable information had higher chances of being acted upon.
Contextual factors, integration into electronic health record systems, and point-of-care presentation also impact decision support systems’ effectiveness.
Organizational culture and workflow are crucial factors in utilizing decision support systems effectively [8, 9,10].
What can we learn from experiences with EDS?
“We think much less than we think we think” – Daniel Kahneman, from the book “Thinking, Fast and Slow.” This quote primarily refers to how humans make decisions and apply them. Behavioral economics highlights the impact of choice presentation on decision-making, with people often favoring the status quo or the first option. In healthcare decision support, presenting options strategically can encourage patients to choose the best option for their health.
The role of choice architecture and digital nudging in EDS
EDS is widely used to aid clinicians in patient care decisions, offering numerous benefits. Overcoming challenges involves ensuring effective decision support in the specific context.
Choice architecture and digital nudging are strategies to influence clinicians’ choices.
However, careful evaluation of expected and unexpected consequences of decision support is crucial.
Future Prospects
Adaptive clinical decision support uses artificial intelligence (AI) to train itself and adapt its algorithms based on new data.
It presents new challenges, such as ensuring safety and effectiveness. Challenges associated with adaptive clinical decision support:
1. Transparency: Clinicians need transparency around the different variables that have gone into making the decision.
2. Potential for biases: It is crucial to ensure that the data used in decision support is accurate and unbiased. Adaptive clinical decision support is an innovative way to incorporate AI in the healthcare industry. It holds great promise, but addressing the challenges associated with this technology is essential.
To ensure the safe and effective use of adaptive clinical decision support, the American Medical Informatics Association has provided broad recommendations. These recommendations cover a wide range of areas, including: Establish processes for overseeing the management of decision support. Monitor the effectiveness of decision support. Educate the workforce about decision support.
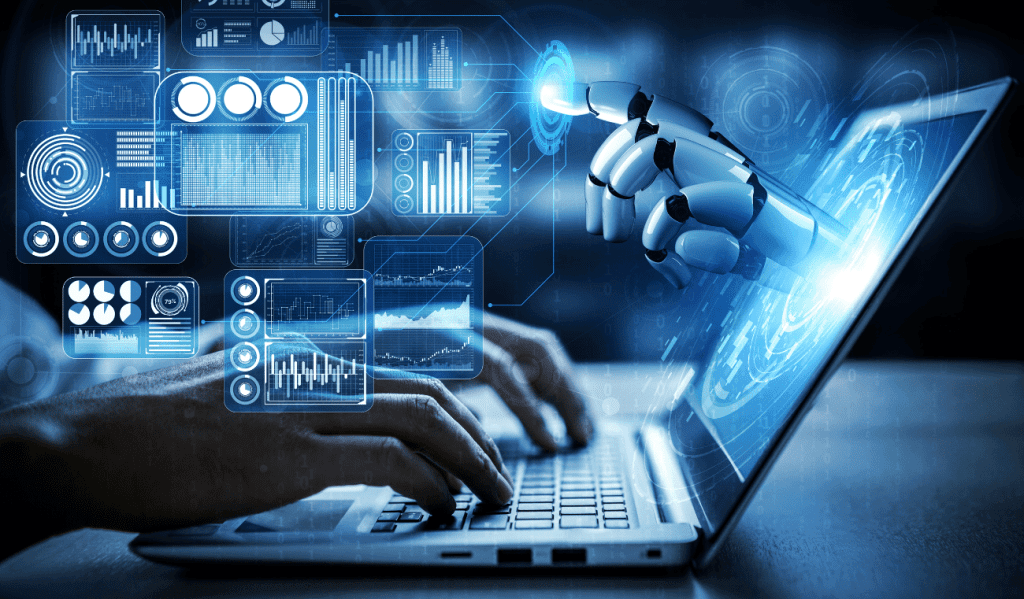
Conclusion
The advancement of artificial intelligence (AI) in healthcare enables efficient and accurate decision-making for patient care. However, effective integration of AI-driven electronic decision support into clinical workflows requires regular testing, refinement, transparency, and rigorous evaluation. Trust-building and transparency are essential for the success of AI-driven decision support systems and to enhance patient care in the healthcare community.
References
- Jaspers, M. W., Smeulers, M., Vermeulen, H., & Peute, L. W. (2011). Journal of the American Medical Informatics Association, 18(3), 327-334
- Varghese, J., Kleine, M., Gessner, S. I., Sandmann, S., & Dugas, M. (2018).Journal of the American Medical Informatics Association, 25(5), 593-602.
- Li, L., Georgiou, A., Vecellio, E., Eigenstetter, A., Toouli, G., Wilson, R., & Westbrook, J. I. (2014, January). HIC (pp. 74-79)
- Camacho, J., Zanoletti-Mannello, M., Landis-Lewis, Z., Kane-Gill, S. L., & Boyce, R. D. (2020). Journal of Medical Internet Research, 22(8), e18388.
- Van Dort, B. A., Zheng, W. Y., Sundar, V., & Baysari, M. T. (2021). Journal of the American Medical Informatics Association, 28(1), 177-183.
- Cordero-Guevara, J. A., Parraza-Díez, N., Vrotsou, K., Machón, M., Orruño, E., Onaindia-Ecenarro, M. J., … & Regalado de los Cobos, J. (2022). BMC Health Services Research, 22(1), 1-13.
- Zheng, W. Y., Richardson, L. C., Li, L., Day, R. O., Westbrook, J. I., & Baysari, M. T. (2018). European journal of clinical pharmacology, 74, 15-27.
- Baysari, M. T., Westbrook, J. I., Richardson, K. L., & Day, R. O. (2011). Journal of the American Medical Informatics Association, 18(6), 754-759.
- Jaensch, S. L., Baysari, M. T., Day, R. O., & Westbrook, J. I. (2013). International Journal of Medical Informatics, 82(10), 980-986.
- Petersen, C., Smith, J., Freimuth, R. R., Goodman, K. W., Jackson, G. P., Kannry, J., … & Wright, A. (2021). Journal of the American Medical Informatics Association, 28(4), 677-684.
Author
-
Director Centre for Health Systems and Safety Research, Australian Institute of Health Innovation Macquarie University, Australia