AI: Interplay among humans, organisations & technology
Read as Magazine PDF
could just mention the basic features of the Danish health care system that is, its universal coverage, tax-based financing, free and equal access, high degree of digitalization and has a lot of problems. But we try to learn from incidents. And more recently, we also tried to learn from excellence. So we have to strike a balance between learning from the negative and then learning from the positive.
AI in health care is improving diagnosis and clinical care, as they said in the report from W.H.O. At the same time, artificial intelligence may change the practice of medicine, increasing the efficiency and accuracy of diagnosis, especially in specialities that rely on imaging such as radiology and pathology. But it’s also been noticed by several that algorithms can underperform when they leave their home hospital and the patient selection. And now the algorithms that I’m going to talk about are mainly exclusively classification algorithms. And here we have several examples that have come up in recent years and they have all been published in and most of them have been published in high-quality journals, actually, and then also in the trade press. So, for instance, Nature Medicine recently wrote about the diagnosis bias of artificial intelligence algorithms applied to chest radiographs in underserved patient populations, and the same groups, the World Economic Forum and Physics World also highlighted this.
Then there was this disturbing news about racists in the machine where it turned out that it was an issue with Google’s labelling. Now, this is being addressed in many forums, for instance, also in the Forbes Forum.
And, when you look up fairness in machine learning on Google Scholar, you find a small score of papers – five, seven years ago. But now within the last two years, it’s almost 2000. So it has the attention to it has risen. Here’s a cartoon from the Danish Medical Daily “Dagens Medicin” where it is quite easy to spot the bias. Here is a pregnant woman and a husband sitting there and the doctor says, I’ll just prescribe something for you. We don’t know how it affects pregnancy, but it has been tested on 10,000 white males. So, therefore, rest assured, you can take it.
RACIAL BIASES FROM MESSY ALGORITHMS
And an example from 2009 is when “Nature” reports that millions of black people are affected by racial bias in health care algorithms. It turned out that the people who were black and white, define themselves as blacks because they were generally assigned lower risk scores than equally sick white people. And the reason why this happened was the data. They were quite representative, but they represented black people having fewer visits to hospitals simply because when they were ill, they didn’t go to hospitals nearly as much as white people. So black patients spent $1,800 less per month in medical costs per year simply because of their circumstances. So this led to the algorithms. Computing incorrectly with the black patients must be healthier since they spend less on health care. This was, of course, a scandal and it was affecting everything that was used to allocate healthcare widely in U.S. hospitals.
Let’s ask about what bias is on if we bias, of course, is a skewness. So it has been used this term and the concept to describe statistical skewness and also in navigation. But the more recent understanding of the use of the term cognitive bias came in the nineties, especially in an extremely influential book called “Judgment Under Uncertainty: Heuristics & Biases” which introduced a rich research program on the study of heuristics and biases that people use when we make decisions under limited information, limited resources, limited time. And the authors introduced several biases and described the categories – confirmation bias, hindsight bias, self-serving bias, anchoring bias, and availability bias. And several books have also described the biases in medical reasoning.
Ironically, prejudice and discrimination are inevitable byproducts of heuristics and biases, namely the efficiency of human cognition. Because if we had to calculate everything in detail, we wouldn’t be able to be effective. It’s interesting to see that this extremely influential book in cognitive psychology is “Thinking Fast and Slow” which came out about a decade ago and also has a lot of citations.
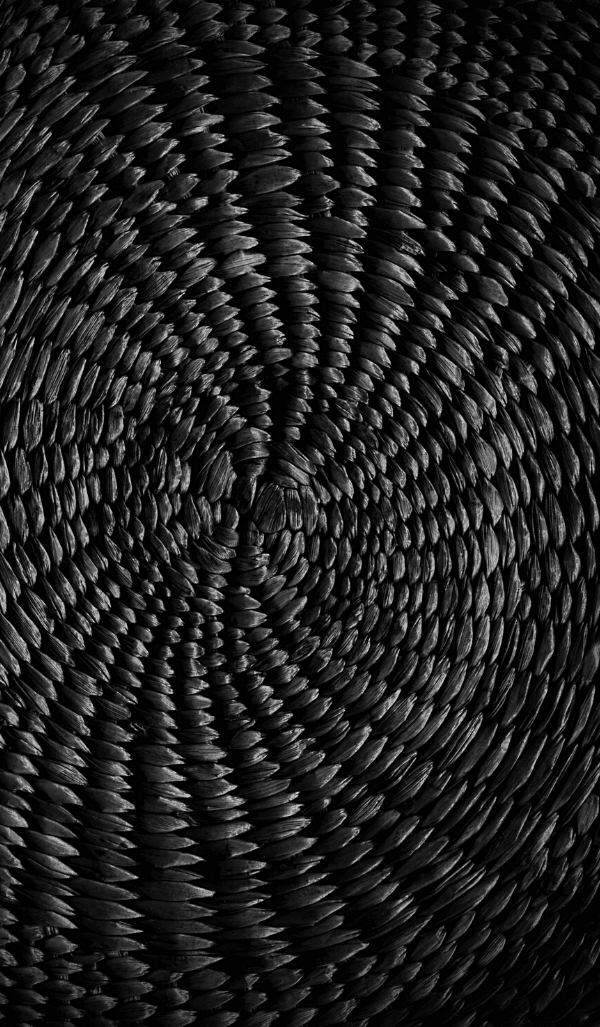
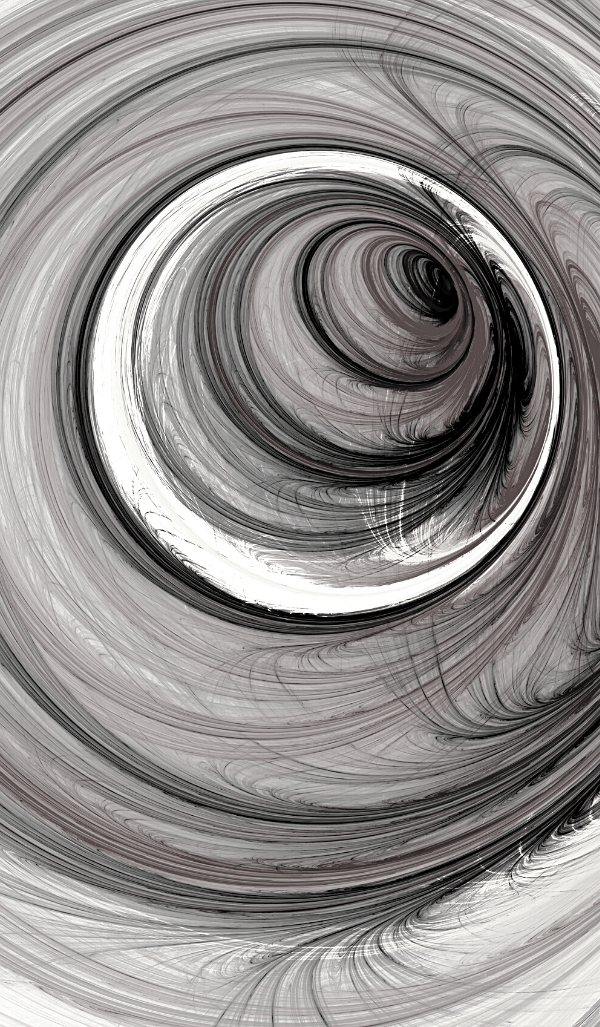
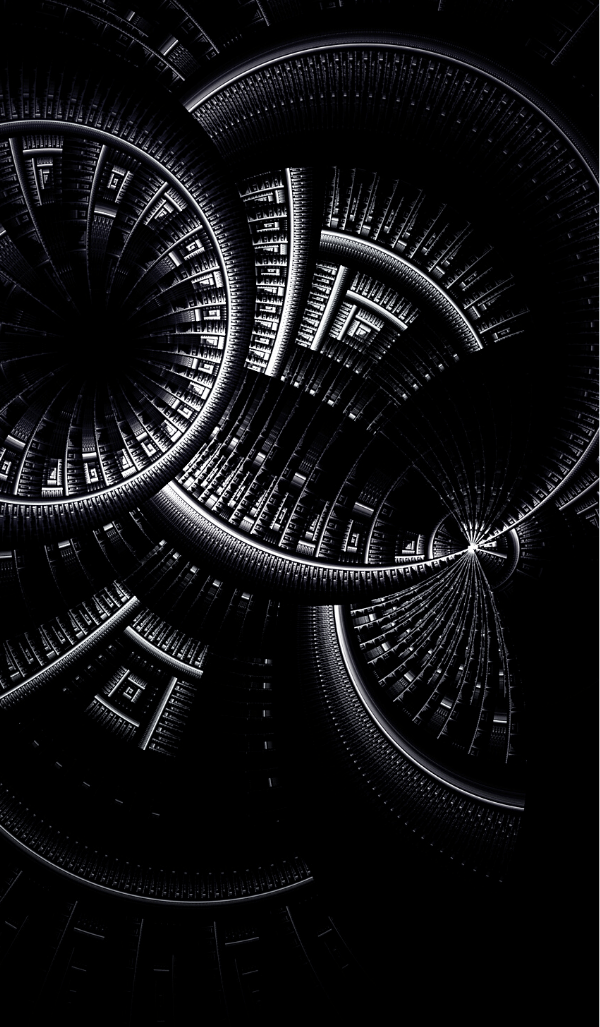
And on the other hand, biases are not only said innocent quality ones – but they are also socially conscious and unconscious.
A bias is an inclination or prejudice for or against one person or group, especially in a way considered unfair.
When we talk about biases and discrimination, it nearly always concerns groups that are vulnerable or in some sense suffering from discrimination and the poor where it is, and I would say a touchy subject. So here we list the attributes that are often cited as requiring bias evaluation, gender, of course, necessity, religion, age, marital status, socio-economic circumstances, disability status, and ability status.
And then actually the European Union’s chapter of fundamental rights extends the protected attributes by mentioning not only gender, race, colour, ethnic or social origin, and genetic justice, but also language, religion, belief, political membership, national minorities, property, birth, disability, aIDS of sexual orientation.
ML PRINCIPLES FOR ACHIEVING FAIRNESS
1 INDEPENDENCE (Demographic Parity)
The proportion of each segment of a protected class (eg: gender, low income) should receive positive outcomes at equal rates.
Limitations: This May result in discrimination against equally eligible/ treatment-needing individuals.
The first one is called independence or demographic parity. Some of the names also really mean that proportion – each segment of a protected class such as gender, low income, etc. To receive positive outcomes at an equal rate as non-protected ones. So the positive outcome is we have them allocated to the outcomes. The limitations, of course, are that they may result in discrimination against equally eligible treatment needing individuals.
2 SEPARATION
The true positive rate and the false positive rate must be equal (and therefore the false negativity rate and the true negative rate are equal) for every value of the sensitive attribute(s).
Limitations: May ignore pre-existing inequality
If we require that the true positive rate and the false positive rate must be equal. So, patients are assigned only after score – independent of gender, and age, and people with a higher survival rate are more likely to get assigned. So again, here we have a limitation that we may ignore preexisting inequalities
3 SUFFICIENCY
Both negative and positive parity has to be achieved (predictive rate parity)
Limitations: Ignore pre-existing inequality among the candidates.
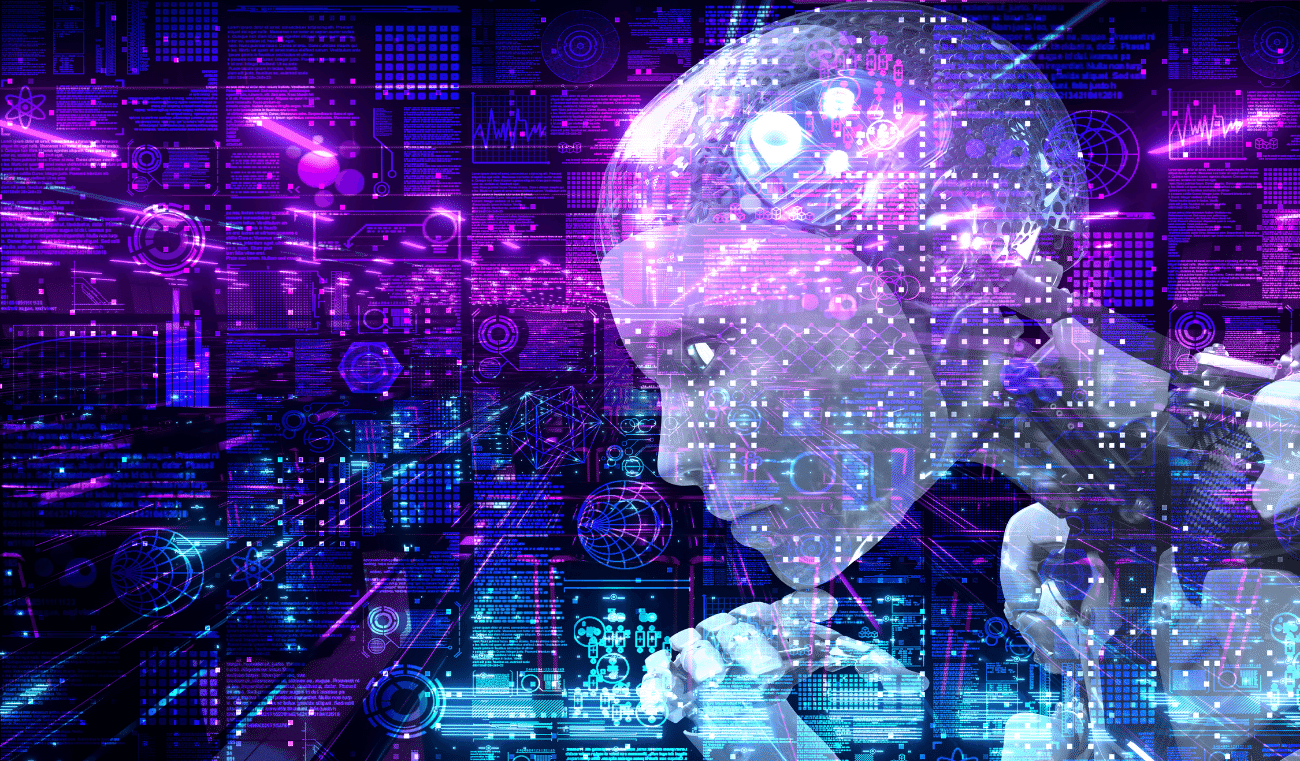
THE IMPOSSIBILITY THEOREM IN ML
No more than one of the three fairness metrics of demographic parity (independence), sufficiency (predictive parity), and separation (equalized odds) can hold at the same time for a well-calibrated classifier and a sensitive attribute capable of introducing machine bias.
We have the impossibility of having an idea, fair and formal principle of fairness. And now I go to review some main ethical principles of fairness that can guide us when we try to regulate the development and deployment of algorithms for sorting patients. The variations of these.
ETHICAL PRINCIPLES OF FAIRNESS
Basic, but incompatible intuitions/principles about fairness:
- Treating “Like Cases” alike
- Ensuring Equal Opportunity
- Compensating for “brute unluck” of any adverse circumstances, seeking to establish a “level playing field”
- Fair distribution based on voluntary transactions
PRINCIPLES OF ETHICS UNDERLYING GOOD PRACTICES IN MEDICINE
TRADITIONAL BIOETHICS PRINCIPLES
- Beneficence
- Non-maleficence
- Autonomy
- Justice
A NEW ENABLING PRINCIPLE
- Explicability
The first one is treating like cases and this seems to be self-evident and harks back for thousands of years to the Greek philosopher Aristotle. Then there’s another one, which is a more recent date, which is about ensuring equal opportunity.
The third one is a sharpening of rules. It is about ensuring, again, equal opportunity by compensating for the lack of any adverse circumstances. And yet the outcome seeks to establish a level playing field. And then finally there is this hard-nosed attitude, namely that health care benefits are fairly distributed if they’re based on voluntary transactions.
So this first principle is about treating cases alike. It’s an example of what has been called a super-thin lenience principle. There can be no ethical ground for providing care to one person and not to another without there being some relevant empirical difference between them (physical or psychological).
Ethics say that you cannot say this act is good, or another other act is not good without having some physical difference. There must be some observable empirical difference. You can’t say that one is good and the other is not good. The same for aesthetic judgments. So if two persons are alike in all relevant attributes, then treating them differently is simply unfair and inconsistent. So there can be no difference in merit or design without some factual difference deserving of care. It’s an ethical property that ‘supervenes’ empirical evidence or attributes.
The second principle that fairness is equal opportunity was published 50 years ago in a major work – Justice as fairness, which is about substantive equality of opportunity. As per this, there are two justice principles. The first one is liberty. Each person has an equal claim to the fully adequate scheme of equal basic rights and liberties, it says. The second one is equality. It suggests that we must have fair equality of opportunity.
If we are perfectly allowed to have unequal treat treatment or distribution of goods, it’s only if they benefit the least advantaged the most. So this is called the Maximin principle of maximizing the minimum.
Then we have a sharpening of this position, which is fairness as an equal opportunity where we compensate – we seek to compensate for any unluck or any adverse circumstances. This, also known as logical determinism, goes further than roles in seeking to compensate for differences in circumstances in which the individual does not influence at all.
Egalitarianism demands that people’s benefits should be determined only by their choice and not by differences in the circumstances. They have no influence.
We cannot have any influence on these issues. Then why should our access to treatment and benefits be dependent on it? Then we have a stop opposite view, the ethical view, namely libertarianism, which holds that all goods, including health care benefits, are fairly distributed if they’re based on voluntary transactions. So society must respect each citizen’s unconditional ownership of themselves and their labour. They also claim that the distribution of goods and health care benefits occurs as a result of voluntary exchange among citizens. So there is little attention to the issues of public utility law to address balanced health care across the population.
So this ethical stance puts an extreme weight on freedom – Liberty.
Author
-
Professor Emeritus, Technical University of Denmark, Department of Management Engineering